Target variables are a significantly important concept in predictive modeling and machine learning. Target variables have a direct effect on the accuracy and effectiveness of machine learning models.
Defining target variables
A target variable is also known as a dependent variable. A target variable is the outcome you aim to predict or explain using your machine learning model. A target variable is the variable that you want to estimate or classify based on the available data.
Target variables in machine learning
Target variables guide the machine learning process. Target variables provide a benchmark for your machine learning model’s performance. You can assess the accuracy and effectiveness of your model by comparing the predicted values to the actual values of the target variable. Target variables serve as the basis for model training. By exposing the model to a large dataset with known target values, the model can learn patterns and relationships.
This enables your machine learning model to make accurate predictions or classifications when faced with unseen data. Choosing your target variable is key. Choosing your target variable determines the type of problem you are trying to solve. It also determines the appropriate algorithms and techniques to use. Different types of target variables require different approaches and considerations.
Different Types of Target Variables
Target variables can take various forms depending on the nature of the problem. These are the different types of target variables:
Categorical variables: Categorical variables represent distinct classes or categories. Categorical variables are used in classification problems. These include predicting whether an email is spam or not. Categorical variables can have two or more classes. Your machine learning model must assign the correct class to each instance based on the available features.
Numerical variables: Numerical variables take on continuous values and are usually used in regression problems. These include stock price predictions, or similar. Your machine learning model must estimate the numerical value of the target variable based on the input features and the patterns observed in the input data and training data.
Ordinal variables: Ordinal variables have a specific order or rank. Ordinal variables are used to solve problems where responses are rated on a scale. Your machine learning model must predict the ordinal value or rank of the target variable based on the available features.
Understanding the nature of your target variable influences how you select your machine learning model. Your target variable affects your machine learning model’s accuracy.
Target variables in predictive modeling
In predictive modeling, target variables are important for enhancing a machine learning model’s accuracy. Target variables are the benchmark for evaluating the performance of your machine learning model. You compare the predicted values to the actual values of the target variable. This assessment tells you about your machine learning model’s accuracy. This assessment also helps you identify areas for improvement, enabling higher levels of accuracy and precision. Target variables also give you insight into the underlying patterns and relationships in the data.
Target variables and accuracy
A predictive model can be used to predict customer churn in a subscription-based service. The target variable would be whether a customer churns or not. By analyzing the relationship between the target variable and customer attributes, we can identify the key factors that contribute to customer churn. This understanding can then be used to implement targeted retention strategies and reduce customer attrition
The effect of incorrect target variables
Picking the wrong target variable messes up your predictions. If it’s not what you’re trying to predict, the model won’t work. If you predict income (a number) as low/medium/high, the model misses details and can’t predict as well. Choosing a clear target variable is key to getting good results from your machine learning model.
How to choose the right target variable
Choosing the right target variable requires careful consideration of several factors. Here are some key points to keep in mind:
- Relevance: Your target variable should be directly related to the problem you are trying to solve. Your target variables should reflect the information or outcome you want to predict or classify.
- Availability: Ensure that you have a sufficient amount of data available that have known target values. A target value is a key metric for you. Without a substantial dataset, your model may struggle to learn meaningful patterns.
- Measurability: The target variable should be measurable. Your target variable should be something that can be classified objectively.
- Balanced Distribution: When you have a categorical target variable, aim for a balanced distribution among the classes. This ensures that your machine learning model is not biased towards a particular outcome
Common mistakes when choosing target variables
Choosing the wrong target variable affects your machine learning model’s accuracy and effectiveness. Avoid these common mistakes when choosing your target variables:
- Choosing an irrelevant target variable that does not provide meaningful insights or predictions.
- Mistaking a derived variable as the target. This leads to circular reasoning and flawed results.
- Ignoring the relationship between the target variable and the input variables. You will miss out on valuable information
Pre-processing target variables
You need to pre-process your target variables before inputting them into your machine learning models. You should handle missing target variables for your predictve model with:
- Imputation: Imputation estimates missing values based on the available data. Common imputation methods include mean imputation and multiple imputation.
- Exclusion: If you have significant amounts of missing data, you may need to exclude those instances from the analysis. Be careful here, to ensure that your data analysis remains representative and unbiased.
- Normalizing: Normalization ensures that the target variable lies within a specific range. This makes it easier for your machine learning model to learn and make accurate predictions.
- Scaling: Scaling adjusts the variance of your target variable. Scalin is useful when dealing with machine learning models that are sensitive to variable scales.
Assessing the performance of target variables in predictive modeling
Assessing the effectiveness of your target variable depends on the type of problem you are trying to solve:
- Classification problems: Accuracy, precision, recall, and F1-score are used metrics to measure the performance of target variables in classification problems.
- Regression Problems: Mean absolute error (MAE), mean squared error (MSE), and R-squared are used to measure the effectiveness of target variables in regression problems.
Improve target variable performance
If you need to improve your target variable’s performance, you can use:
- Feature engineering: Create new derived features from existing data. That may enable you to discover additional predictive power in the target variable.
- Data augmentation: Increase the size and variety of the dataset to expose your machine learning model to a wider range of patterns.
- Model selection and optimization: Experiment with different machine models and fine-tune your hyperparameters
So, there you have it! A comprehensive understanding of target variables in machine learning. Remember, the choice and proper preprocessing of a target variable are critical for accurate predictions and effective model performance.
Graphite Note is here to help you harness the power of machine learning without the need for complex coding. Whether you’re part of a growth-focused team, an agency without a data science department, or a data analyst looking to delve into AI, our platform is designed to transform your data into precise predictions and actionable strategies with just a few clicks. Experience the synergy of our no-code predictive analytics tools and see how easy it is to predict business outcomes and make data-driven decisions.
Request a Demo today and take the first step towards unlocking unparalleled insights and efficiency for your business.
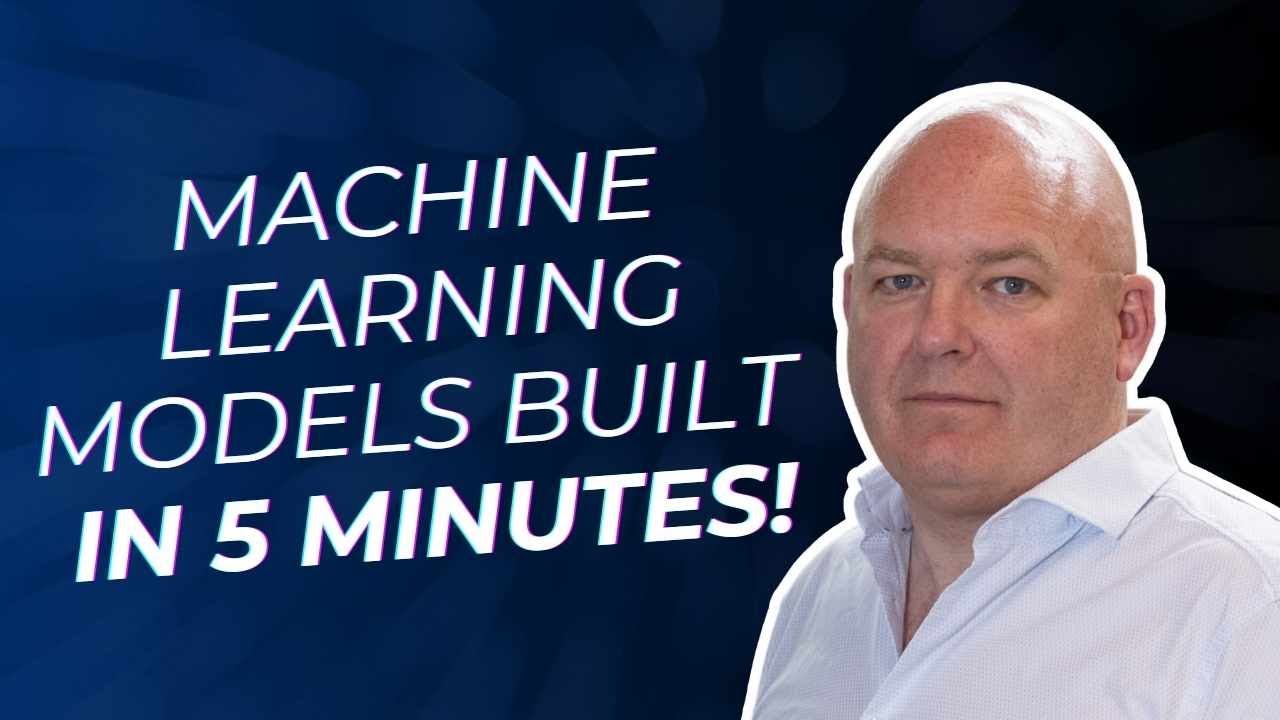
Transform your business with Graphite Note’s prebuilt machine learning models that work with any dataset, regardless of industry. Our data-agnostic platform delivers insights and predictions in minutes, giving you a competitive edge. Explore high-impact use cases that drive real business outcomes:
Key Use Cases:
1. Lead Scoring: Prioritize high-quality leads and increase conversion rates. Customer Churn Prevention: Retain more customers by identifying those at risk of churning.
2. Cross-Sell Optimization: Boost sales by targeting customers likely to purchase additional products.
3. Time Series Forecasting: Optimize inventory and plan for sales peaks and low periods.
4. Customer & Product Segmentation: Segment customers for personalized strategies and sales growth.
5. Customer Lifetime Value: Predict customer value over time to maximize revenue.
6. Predictive Maintenance: Reduce downtime, cut costs, and extend machinery life. Start leveraging Graphite Note today for smarter decisions and faster results!
Try it for free and see how predictive and prescriptive analytics can revolutionize your strategy.