What is Predictive Analytics? A Definition and Overview
Predictive analytics is a powerful tool. Predictive analytics help you make better data-driven decisions. Predictive analytics uses statistical algorithms and machine learning techniques. Predictive analytics enables you to forecast future outcomes and trends. Your forecasts from predictive analytics are based on historical data. Predictive analytics goes beyond descriptive analytics. Predictive analytics enables you to make proactive decisions to drive business growth.
Understanding predictive analytics
Predictive analytics uses data to make predictions about future events or behaviors. Predictive analytics analyzes historical data. Predictive analytics then identifies patterns, trends, and relationships. These are used to create models that can predict future outcomes. You can use your predictive models to optimize your business operations. You can also use your predictive models to improve customer satisfaction. You can also use predictive models to drive revenue growth.
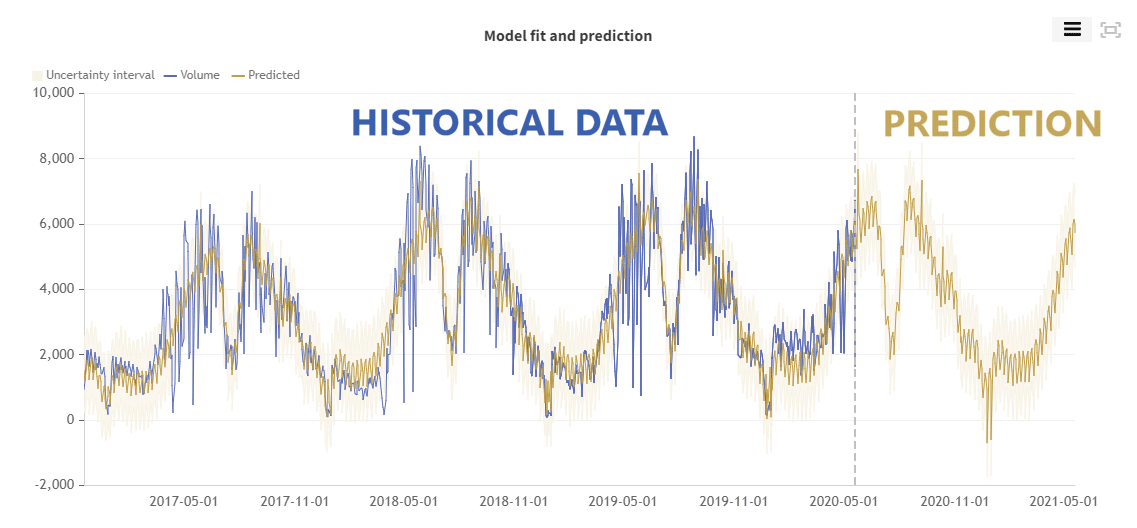
What is predictive analytics?
Predictive analytics focuses on the valuable insights you can glean from historical data. Predictive analytics uses historical data to predict future outcomes. Predictive analytics uses data mining and advanced statistical techniques. Predictive analytics helps you make data-driven decisions for your business. Predictive analytics gives you actionable insights for your business. Predictive analytics can help you grow your profit margins.
Machine Learning algorithms
Machine learning leverages algorithms to train machines on large datasets. AI technology enables the machines to make informed decisions. These decisions are based on patterns and correlations. Machine learning algorithms are designed to analyze large amounts of data. Through this analysis, machine learning algorithms identify patterns. The machine learning algorithms then make predictions or decisions based on those patterns.
Data visualization
Predictive analytics tools use data visualization techniques. Predictive analytics software presents data in a visual format. This makes it easy to understand and interpret complex patterns and trends.
Importance of predictive analytics for your business
Predictive analytics gives your business a competitive edge
Predictive analytics give your business a competitive edge. In marketing, for example, predictive analytics can help you optimize your campaigns. Predictive analytics lets you analyze customer data and behavior. From there, you can personalize marketing campaigns and target customer segments.
Predictive analytics and supply chain management
Predictive analytics is useful for supply chain management. Predictive analytics analyzes historical data to help you optimize your supply chain. You can optimize your inventory levels using predictive analytics. You can improve demand forecasting, and streamline your logistics operations. This helps you cut back on costs and ensure customer satisfaction.
How do predictive analytics work?
Predictive analytics has four main steps:
Data Collection
Data collection involves gathering relevant data from various sources. You could gather data from various sources. These include: customer databases, transaction records, social media interactions, and sensor data. The more diverse and comprehensive the data, the better the predictions are likely to be. During data collection, it’s important to ensure data quality and compatibility. You will need to undertake data cleaning. Data cleaning removes any errors or inconsistencies. During data cleaning, you also set out the data in a format that’s easy to analyze. Data cleansing techniques include removing duplicates, handling missing values, and standardizing data formats. Data privacy and security are also important during this phase. Ensure you adhere to privacy regulations and implement robust security measures.
Data Preparation
Data preparation is where your raw data is set out in a suitable format for predictive analytics models. Data preparation includes a range of tasks. These include: data cleaning, data integration, feature engineering, and data normalization. Data cleaning involves removing any outliers, inconsistencies, or errors in the data. Data integration involves combining data from different sources into a unified dataset. Feature engineering is the process of creating new features or transforming existing features. Data normalization is the process of scaling the data to a common range or distribution.
Model Building
Model building is the heart of predictive analytics. Model building involves selecting and applying statistical algorithms and machine learning techniques. Your goal is to build an effective predictive model. An effective predictive model learns from historical data. An effective predictive model then makes accurate predictions on new, unseen data. Your choice of predictive modeling technique will depend on the nature of the problem you’re trying to solve. Your predictive modeling choice is also determined by your available data. You will choose a model based on your desired level of accuracy. During model building, you will split your data into training data sets and testing data sets.
Training data is used to train your predictive analytics model. Testing data is used to evaluate your predictive analytics model’s performance. During model building, you will also set and tune parameters.
Parameter tuning involves testing different configurations of your model. You may use various techniques, like cross-validation or grid search.
Model Deployment
Model deployment involves integrating your predictive models into your business systems or processes. From there, you will be able to make real-time predictions or periodic forecasts. Your deployment strategy depends on your specific business needs and requirements. You can integrate your predictive analytics model into a web application.
You could integrate your model into a mobile application. You could deploy your model as part of a batch process. Once you have deployed your model, you must continue to monitor its performance. You should track your model’s accuracy, and refine it as new data becomes available. Current data keeps your predictive analytics system effective and up-to-date. Current data can be used to further fine-tune your predictive model.
What are the key components of predictive analytics?
Predictive analytics techniques have four key components:
- Data mining: Data mining is the process of extracting valuable insights and patterns from large datasets. Data mining involves several techniques. These include: clustering, classification, association rule mining, and anomaly detection. Data mining helps to uncover hidden patterns and relationships in your data. These can be used to make predictions.
- Statistical modeling: Statistical modeling involves applying statistical techniques. These are used to identify relationships and correlations in the data. This includes techniques such as regression models, regression analysis, time series analysis, and hypothesis testing. Statistical modeling helps to quantify the effect of different variables on the outcomes. Statistical modeling helps you assess the statistical significance of the predictions.
- Machine Learning algorithms: Machine learning algorithms enable computers to learn from data and make predictions. Machine learning techniques include supervised learning, unsupervised learning, and reinforcement learning. These algorithms can handle complex patterns and non-linear relationships in your data. Machine learning algorithms are well-suited for predictive analytics tasks.
- Data visualization: Data visualization enables you to enjoy graphical representations of your data and predictions. Visualization techniques include charts, graphs, and heatmaps. These visuals help you to communicate complex patterns and trends. Data visualization enables you to better understand the insights generated by your models. Data visualization helps you make better decisions.
Types of Predictive Analytics
There are three main types of predictive analytics:
- Descriptive Analytics: Descriptive analytics summarizes historical data. Descriptive analytics then provides insights into what has happened in the past. Descriptive analytics enables you to understand trends, patterns, and correlations in your data. Descriptive analytics is the foundation of predictive analytics. Descriptive analytics provides the historical data needed to build predictive models.
- Diagnostic Analytics: Diagnostic analytics aims to understand why certain events or outcomes occurred. Diagnostic analytics involves a detailed analysis of historical data. Diagnostic analytics uncover the root causes of specific events or trends.
- Prescriptive Analytics: Prescriptive analytics gives you recommendations and actions to optimize future outcomes. Prescriptive analytics combines historical data, predictive models, and business rules. Prescriptive analytics helps you make data-driven decisions. Prescriptive analytics helps you take proactive measures to achieve your goals.
Predictive analytics in business
Predictive analytics can help to improve customer satisfaction. Predictive analytics can help you increase sales. Predictive analytics can guide you towards optimizing your supply chain. Predictive analytics can analyze customer data to help you personalize marketing campaigns. You can use predictive analytics to build product recommendations for your customers. You can improve your customer service using predictive analytics. Predictive analytics in demand forecasting helps you optimize inventory levels and reduce costs.
Predictive analytics in healthcare
Predictive analytics is used in healthcare. Predictive analytics helps medical professionals identify patients at risk of developing diseases. Predictive analytics analyzes patient data and medical records to do so. This helps medical professionals take a proactive approach to patient care. An enhanced patient care approach reduces hospital readmissions and lowers healthcare expenses.
Predictive analytics in finance
Predictive analytics can be used to assess customer credit risk. Predictive analytics can also detect fraudulent activities, and help you make investment decisions. Predictive models can help financial institutions make informed decisions. Predictive analytics is also used in investment portfolio management.
What are the challenges associated with predictive analytics?
Various factors can affect the effectiveness of your predictive analytics models. These include:
Data quality and data management: The effectiveness of your predictive analytics relies heavily on the data you feed it. Data cannot be incomplete, inconsistent, or biased. If your data is not of good quality, the predictive model will generate misleading or inaccurate predictions.
Ethical and privacy concerns: Predictive analytics can use sensitive data. It is vitally important to ensure you adhere to data privacy regulations. Your data repository and systems must be well-secured to prevent unauthorized access.
Conclusion
Leveraging predictive analytics can bolster your business and decision making processes.