Machine learning trends are taking the tech world by storm, and 2025 promises to be an exciting year for breakthroughs in everything from data analysis to real-time applications. As the founder of Graphite Note, I’ve seen firsthand how these developments have accelerated the adoption of no-code solutions in predictive analytics, revolutionizing the way organizations handle data.
In this post, we’ll explore ten key directions shaping the future of machine learning. By the end, you’ll walk away with a deeper understanding of how these trends can drive innovation, sharpen decision-making processes, and open doors to new opportunities in your organization.
Democratization of ML Through No-Code Platforms
Machine learning is no longer the sole territory of PhDs and data scientists. The rise of no-code platforms is allowing business professionals, marketers, and even educators to unlock the power of ML without extensive programming skills. These platforms leverage intuitive interfaces, automated workflows, and prebuilt templates to make predictive analytics widely accessible. According to a Gartner report, no-code/low-code solutions could account for 70% of new applications developed by 2025, underscoring the magnitude of this shift.
Key benefits of no-code ML tools include:
- Faster time to market, as development cycles are drastically reduced
- Reduced reliance on niche experts, lowering operational costs
- Greater flexibility for domain experts to create tailored models
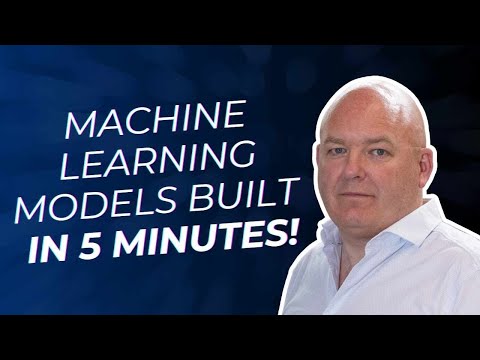
Real-Time Data Processing and Edge Computing
As connected devices multiply, real-time data processing becomes more critical. Traditional cloud-based ML solutions can struggle with latency, leading to delays when milliseconds matter. Edge computing solves this by processing data closer to the source—on local hardware or on the device itself—to minimize round trips to a remote server.
Why this matters:
- Healthcare devices can perform diagnostic scans on the spot
- Autonomous vehicles can make split-second decisions
- Retailers can deliver on-the-fly personalized recommendations
Look for 2025 to bring a surge in edge computing advancements, complementing machine learning trends that focus on immediate data processing and real-time analytics.
Ethical and Responsible AI
With machine learning’s growing influence comes a heightened need for transparency, fairness, and accountability in predictive models. Inaccurate algorithms can inadvertently harm marginalized communities or reinforce biases in hiring decisions. Policymakers and industry leaders alike are calling for clear guidelines and regulations.
Steps toward responsible AI:
- Implement rigorous bias detection protocols
- Use diverse training datasets to reduce skewed results
- Enhance model interpretability, so stakeholders understand the “why” behind predictions
Expect more robust frameworks and best practices to emerge around ethical AI development, such as explainable models that reveal how input data generates specific outcomes.
Advanced Natural Language Processing (NLP)
Natural language processing has made leaps in recent years, but 2025 will usher in even more impressive language models. These advanced algorithms can interpret context, tone, and nuances in text, making them invaluable for customer service, social media insights, and content generation.
Key NLP innovations to watch:
- Sentiment analysis that captures emotional subtleties
- Multilingual support with near-instant translation
- Context-aware chatbots for personalized user experiences
Organizations that integrate NLP into their workflows can transform user interactions and gather richer insights from unstructured text data.
Automated Feature Engineering
Feature engineering is at the heart of building robust machine learning models. Yet, it often demands significant domain expertise and time-consuming trial and error. By 2025, we can expect automated feature engineering to take center stage among machine learning trends, making it simpler for teams to identify optimal predictors with minimal human intervention.
- Time savings: Automated algorithms can systematically generate, select, and transform variables faster than manual processes.
- Consistency: Data scientists and no-code users can rely on reproducible techniques, reducing model bias and errors.
- Scalability: As organizations accumulate more data, automated feature engineering tools help maintain model accuracy without overwhelming ML teams.
In combination with no-code ML platforms like Graphite Note, automated feature engineering removes guesswork and streamlines predictive analytics for users of varied backgrounds.
Federated Learning Gains Momentum
As data privacy regulations get stricter, federated learning stands out as a method for training models without centralizing raw data. Instead of sending datasets to a single server, the model travels to different nodes—like smartphones or local servers—and learns from distributed data.
Why this approach is poised for growth:
- Privacy preservation: Sensitive information never leaves the user’s device, reducing compliance risks.
- Reduced bandwidth: Uploading massive datasets to the cloud becomes unnecessary, lowering operational costs.
- Collaborative potential: Multiple organizations can pool insights without sharing proprietary data.
Federated learning dovetails with other machine learning trends—like edge computing—by running computations closer to data sources, ensuring rapid, secure results.
Industry-Specific Solutions
One-size-fits-all approaches to machine learning are losing ground to highly specialized solutions. From healthcare diagnostics to financial fraud detection, verticalized tools focus on niche data types, terminologies, and workflows. These targeted platforms empower users to produce higher-quality outcomes without wrestling with generic algorithms.
Examples of specialized solutions:
- Healthcare: Models trained on medical images for faster diagnoses
- Retail: Sales forecasting systems that optimize supply chain decisions
- Manufacturing: Predictive maintenance to prevent costly equipment failures
By honing in on domain-specific complexities, these solutions maximize accuracy and relevance.
Reinforcement Learning for Strategic Decision-Making
Reinforcement learning (RL) has matured, shifting from game-based achievements (like beating humans at Go) to real-world applications in robotics and resource allocation. In 2025, RL is expected to tackle increasingly sophisticated challenges, from optimizing warehouse operations to managing energy distribution grids.
Core RL benefits:
- Decision-making that continuously adapts based on new information
- Ability to learn from failures in simulated environments before deployment
- Empowerment for systems to respond dynamically to changes in conditions
Organizations adopting RL can build automated systems that refine their strategies over time, driving better productivity and lower costs.
MLOps and Continuous Monitoring
Just as DevOps transformed software development, MLOps (Machine Learning Operations) is changing the way ML models move from inception to production. By incorporating best practices around version control, monitoring, and continuous integration, MLOps ensures that models remain accurate and up to date.
Essential MLOps components:
- Model versioning: Tracking every iteration of a model’s code and dataset
- Automated retraining: Regularly updating models as new data appears
- Performance dashboards: Monitoring predictions in real time to catch anomalies
According to a recent McKinsey & Company article, disciplined MLOps practices can drastically cut the time it takes to deploy updates while minimizing failures.
Hybrid AI Approaches and AI Agents
Finally, hybrid AI merges different techniques—like combining rule-based systems with neural networks—to overcome the weaknesses of any single methodology. By fusing symbolic reasoning with machine learning, for example, hybrid models can interpret data more intuitively, making them suitable for complex sectors like law, finance, and scientific research.
Hybrid AI in action:
- Automated contract review: Blending semantic understanding of legal clauses with predictive analytics
- Financial risk assessment: Integrating conventional scoring rules with deep learning methods
- Scientific breakthroughs: Harnessing symbolic logic to interpret novel data in physics or biology
These blended approaches round out our list of top machine learning trends for 2025, highlighting the creative solutions that emerge when AI disciplines intersect.
Final Thoughts on the Future of Machine Learning
The potential for machine learning has never been more apparent. Innovations in no-code ML, real-time data processing, automated feature engineering, and responsible AI are driving this discipline forward at breakneck speed. As a founder at Graphite Note, I’ve witnessed an increasing demand for user-friendly platforms that bring predictive analytics to everyone, not just those with advanced technical skills.
From developing ethical guidelines to embracing federated learning, these machine learning trends underscore an industry-wide focus on scalability, transparency, and collaboration. By staying informed and integrating these technologies thoughtfully, you can ensure your organization remains agile and ready to capitalize on the transformative power of ML.