Predictive analytics has emerged as a powerful tool for businesses looking to unlock growth opportunities. With the ability to harness insights from past data, organizations can make informed decisions and steer their operations in the right direction. However, many companies shy away from utilizing predictive analytics due to the perceived need for AI expertise. The good news is, you don’t have to be an AI expert to leverage the power of predictive analytics for your business. In this article, we will explore nine approaches to help you leverage predictive analytics for growth without the need for AI expertise.
Understanding Predictive Analytics
Predictive analytics is the practice of using historical data to make predictions about future events or trends. By analyzing patterns and identifying correlations, businesses can gain valuable insights and make informed decisions. Let’s start by understanding the basics of predictive analytics.
Predictive analytics involves four key steps: data collection, data preparation, model development, and deployment. First, businesses must collect relevant data from various sources. This can include customer data, sales figures, marketing campaigns, social media interactions, and more. The more diverse and comprehensive the data, the more accurate the predictions will be. Once the data is collected, it needs to be prepared and cleaned to ensure accuracy. This involves removing any outliers, filling in missing values, and standardizing the data.
After the data is prepared, statistical models are developed to analyze the data and generate predictions. These models can range from simple linear regression models to more complex machine learning algorithms. The choice of model depends on the nature of the data and the specific problem being addressed. The models are trained using historical data, and their performance is evaluated using various metrics such as accuracy, precision, and recall.
Finally, these models are deployed to drive business decisions. The predictions generated by the models can be used to optimize various aspects of the business. For example, predictive analytics can help businesses forecast demand, identify potential churners, personalize marketing campaigns, optimize pricing strategies, and improve supply chain management. By leveraging the power of predictive analytics, businesses can gain a competitive edge in the market.
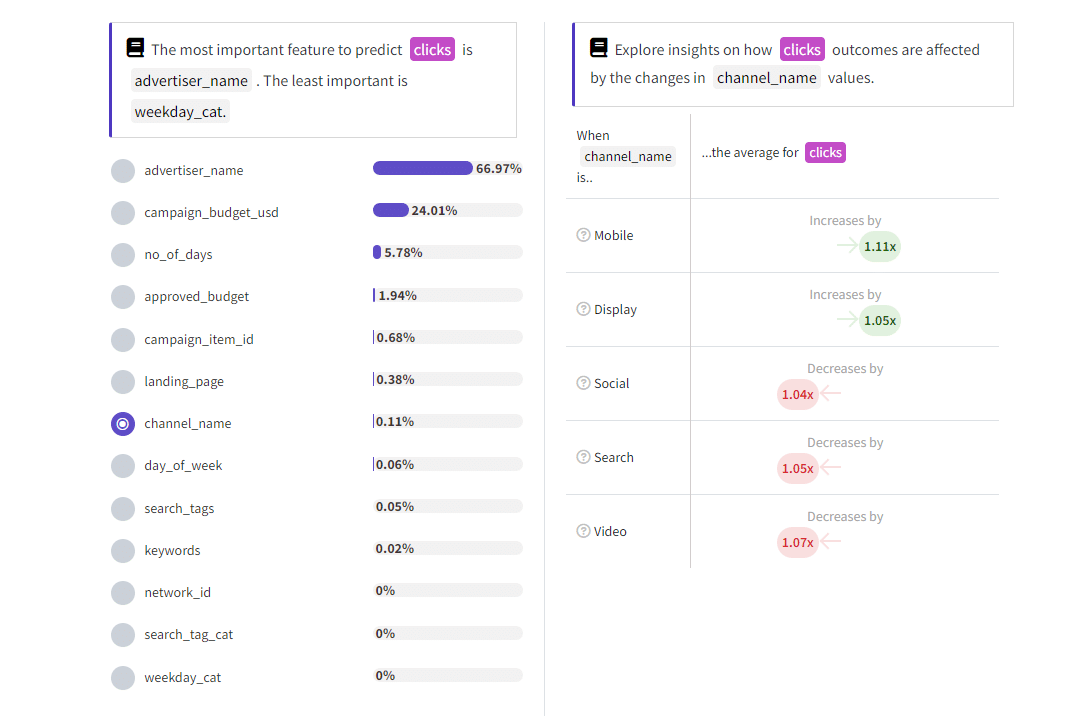
The Role of Predictive Analytics in Business Growth
Predictive analytics plays a crucial role in driving business growth. By leveraging historical data to make accurate predictions, businesses can identify trends, anticipate customer needs, optimize operations, and mitigate risks. For instance, by analyzing customer data, businesses can identify patterns and preferences, allowing them to offer personalized recommendations and improve customer satisfaction. This, in turn, leads to increased customer loyalty and retention.
Predictive analytics also helps businesses optimize their operations. By analyzing historical sales data, businesses can forecast demand and optimize inventory management. This ensures that the right products are available at the right time, minimizing stockouts and reducing carrying costs. Additionally, predictive analytics can help identify potential bottlenecks in the supply chain and optimize logistics, leading to improved efficiency and cost savings.
Furthermore, predictive analytics enables businesses to mitigate risks. By analyzing historical data and identifying patterns, businesses can predict potential risks and take proactive measures to mitigate them. For example, predictive analytics can help identify fraudulent transactions, detect anomalies in network traffic, and predict equipment failures. By addressing these risks before they occur, businesses can minimize losses and maintain business continuity.
In conclusion, predictive analytics is a powerful tool that allows businesses to make data-driven decisions and drive business growth. By leveraging historical data and advanced statistical models, businesses can gain valuable insights, optimize operations, and mitigate risks. As technology continues to advance, the potential of predictive analytics will only grow, enabling businesses to stay ahead in an increasingly competitive market.
The Need for AI Expertise in Predictive Analytics
While predictive analytics offers immense potential for growth, many companies hesitate to adopt it due to the misconception that AI expertise is a prerequisite. Let’s debunk this myth and explore the need for AI expertise in predictive analytics.
Predictive analytics is the practice of using historical data and statistical algorithms to make predictions about future events or outcomes. It allows businesses to gain valuable insights and make informed decisions based on data-driven analysis. However, there are common misconceptions surrounding the role of AI in predictive analytics.
Common Misconceptions About AI and Predictive Analytics
One common misconception is that AI expertise is required to develop and deploy predictive analytics models. While AI can enhance predictive analytics, it is not a requirement. Predictive analytics can be achieved using simpler statistical models and tools that do not necessitate AI expertise.
For example, linear regression is a widely used statistical technique that can be employed to predict future outcomes based on historical data. It does not involve complex AI algorithms but can still provide valuable insights for businesses.
Another misconception is the belief that only large organizations can afford the technology and resources needed for predictive analytics. Fortunately, there are cost-effective solutions available that can be tailored to the needs and budgets of businesses of all sizes.
Cloud-based platforms and software-as-a-service (SaaS) solutions have made predictive analytics more accessible to small and medium-sized enterprises. These platforms offer pre-built models and tools that can be easily implemented without extensive AI expertise.
Overcoming the AI Expertise Barrier
So, how can businesses overcome the AI expertise barrier and leverage predictive analytics for growth? One approach is to utilize pre-built analytic models. These models are developed by experts in the field and can be customized to suit specific business needs.
By leveraging pre-built models, businesses can save time and resources that would otherwise be spent on developing complex AI algorithms. These models can be easily integrated into existing systems and workflows, allowing companies to quickly start reaping the benefits of predictive analytics.
In addition, businesses can also consider partnering with AI consulting firms or hiring data scientists with expertise in predictive analytics. These professionals can provide guidance and support in implementing and optimizing predictive analytics models.
Furthermore, investing in employee training and development programs can help bridge the AI expertise gap within organizations. By providing employees with the necessary skills and knowledge, businesses can build an internal team capable of effectively utilizing predictive analytics tools and techniques.
In conclusion, while AI expertise can certainly enhance predictive analytics, it is not a prerequisite for its successful implementation. Businesses of all sizes can leverage predictive analytics to gain valuable insights and drive growth, even without extensive AI knowledge. By debunking the misconceptions surrounding AI and predictive analytics, companies can embrace this powerful tool and unlock its full potential.
Approach 1: Utilizing Pre-Built Analytic Models
Pre-built analytic models provide businesses with ready-made solutions to leverage predictive analytics. These models have already undergone rigorous testing and fine-tuning, making them reliable and accurate. Let’s explore the benefits of utilizing pre-built models and how to select the right one for your business.
Benefits of Pre-Built Models
Utilizing pre-built models saves businesses time and effort in model development. It eliminates the need for AI expertise and allows organizations to focus on using the insights generated by the models for decision-making. This can be particularly advantageous for small businesses or those without dedicated data science teams.
One of the key benefits of pre-built models is their reliability and accuracy. These models have been developed by experts in the field who have fine-tuned them to ensure optimal performance. By leveraging these pre-built models, businesses can have confidence in the predictions and insights generated, enabling them to make informed decisions.
Furthermore, pre-built models are often backed by a team of experts who continuously update and improve them. This means that businesses can benefit from ongoing enhancements and advancements in predictive analytics without having to invest additional resources. The continuous improvement of pre-built models ensures that businesses are always utilizing the latest techniques and methodologies.
Selecting the Right Pre-Built Model
When selecting a pre-built model, businesses should consider the specific needs and goals of their organization. It’s important to choose a model that aligns with your industry, data requirements, and the type of predictions you want to make.
Conducting thorough research is crucial in selecting the right pre-built model. Businesses should explore the available options, compare their features and capabilities, and read reviews from other organizations that have used the models. This research will help businesses gain insights into the strengths and limitations of each pre-built model, allowing them to make an informed decision.
Consulting with experts in the field can also be beneficial when selecting a pre-built model. These experts can provide valuable guidance and advice based on their experience and knowledge. They can help businesses understand the technical aspects of the models and assess their suitability for specific use cases.
Ultimately, selecting the right pre-built model is crucial for businesses to maximize the value of predictive analytics. By choosing a model that aligns with their needs and goals, businesses can leverage the power of pre-built models to drive growth and make data-driven decisions.
Approach 2: Leveraging Open Source Tools
Another approach to leveraging predictive analytics without AI expertise is to utilize open source tools. These tools provide businesses with the flexibility to customize and adapt the analytics to suit their specific needs. Let’s dive into the world of open source tools and how they can maximize the power of predictive analytics.
Introduction to Open Source Tools
Open source tools are software programs or frameworks that are freely available for anyone to use, modify, and distribute. They offer a cost-effective alternative to proprietary software and provide businesses with the freedom to customize and extend the capabilities of the tools to meet their unique requirements.
One popular open source tool is R, a programming language and software environment for statistical computing and graphics. With R, businesses can leverage a vast array of statistical and graphical techniques to analyze and visualize their data. R’s extensive library of packages allows users to perform various predictive analytics tasks, such as regression analysis, time series forecasting, and machine learning.
Another widely used open source tool is Python, a versatile programming language that offers a rich ecosystem of libraries and frameworks for data analysis and machine learning. With libraries like scikit-learn, TensorFlow, and PyTorch, businesses can build and deploy sophisticated predictive models without the need for AI expertise.
Maximizing Open Source Tools for Predictive Analytics
Open source tools like Graphite Note provide a user-friendly interface and intuitive workflows that allow businesses to harness the power of predictive analytics without AI expertise. With Graphite Note’s no-code approach, users can easily import their data, select the appropriate analytics models, and generate valuable insights. Empowered with these insights, businesses can make data-driven decisions and propel their growth without the need for AI expertise or expensive resources.
Another open source tool that deserves mention is Apache Spark, a fast and general-purpose cluster computing system. Spark’s machine learning library, MLlib, provides a scalable and distributed framework for building predictive models. With its ability to process large volumes of data in parallel, Spark enables businesses to perform advanced analytics tasks efficiently.
In addition to these tools, open source platforms like Hadoop and Apache Kafka play a crucial role in enabling businesses to store, process, and analyze massive amounts of data. By leveraging these platforms, businesses can build robust data pipelines and perform real-time analytics, opening up new possibilities for predictive analytics.
Furthermore, open source communities foster collaboration and knowledge sharing among developers and data scientists. These communities provide forums, mailing lists, and online resources where users can seek help, share ideas, and contribute to the development of open source tools. The collective effort of these communities ensures that open source tools continue to evolve and improve, benefiting businesses of all sizes.
Approach 3: Partnering with Analytics Service Providers
For businesses that prefer a hands-off approach, partnering with analytics service providers is an excellent option to leverage predictive analytics. Let’s explore the value of analytics partnerships and how to choose the right service provider.
The Value of Analytics Partnerships
Analytics service providers bring extensive expertise and resources to the table, allowing businesses to tap into the power of predictive analytics without the need for in-house AI expertise. These partnerships provide access to cutting-edge technologies and a team of experts who can tailor analytics solutions to meet the specific needs of your business. By partnering with an analytics service provider, businesses can accelerate their growth journey and gain a competitive edge.
Choosing the Right Analytics Service Provider
When selecting an analytics service provider, it’s important to consider their expertise, track record, and the industries they specialize in. Look for providers who have a proven track record of delivering successful analytics solutions and have a deep understanding of your industry. Additionally, consider their scalability and flexibility to adapt to your evolving needs. By choosing the right analytics service provider, you can unlock the full potential of predictive analytics and achieve remarkable growth for your business.
With the rise of predictive analytics, businesses no longer need to be AI experts to leverage its power for growth. By utilizing pre-built analytic models, leveraging open source tools like Graphite Note, or partnering with analytics service providers, organizations can harness the insights hidden within their data and make data-driven decisions that propel their growth. So don’t let the misconception of needing AI expertise hold you back – embrace the power of predictive analytics and unlock new horizons of growth for your business!